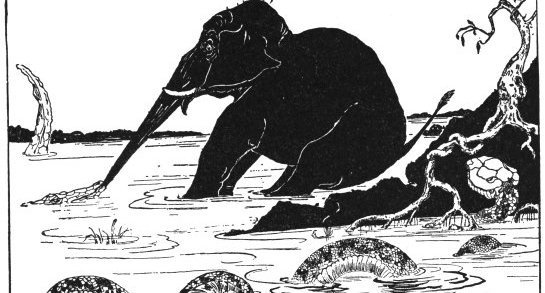
Us humans love a story that has a nice beginning, some events in the middle and a positive end. And the people who are trying to sell us something know this all too well. These are known as “just so” stories. Everything you need to know about the outcome is assumed to be contained in the story. Here’s an example:
“Company X was losing customers at an ever increasing rate. The CIO and COO got together and decided to create a Data Lake using [place your technology/software here] to analyse all of the various disparate data sources in a single repository. They also hired a Data Scientist who used the technology to identify a cohort of at-risk customers. A churn prevention program was developed to target the at-risk group and Company X recovered its lost ground and began to grow its customer base again.”
The implication is that “if your business has customer churn issues then you should consider talking to [place your vendor here].”
The problem is that the reality of the above use case is probably a lot messier than the just-so story leads us to believe. This is the far more likely scenario:
“The CIO of Company X hired a Data Scientist who managed to obtain some IT resources out of the datawarehousing team to germinate an unstructured data repository. The Data Scientist, as a side project, poured data from multiple systems into the new repository. The Data Scientists official project was developing some scorecards and dashboards for the COO, based on some updated efficiency KPIs. As a result of building the dashboards, the Data Scientist became aware that churn was high and, on a hunch, decided to hit the new repository to see if there were any unique characteristics of the churning customers. The Data Scientist happened to get hold of an unused license of [vendor product here] to do the analysis. Turned out that there were indeed unique characteristics of churning customers, and the Data Scientist proudly told the CIO and COO about it….etc”.
Firstly the CIO’s reason for hiring the Data Scientist was not deliberately to tackle customer churn. Also it was the Data Scientist who managed to, almost covertly, divert IT resources to create the embryonic Data Lake. So investment in a Data Lake was not a deliberate decision by executive trying to solve a particular business problem. The Data Scientist could easily have looked into the unique characteristics of the churning customers and not found any, simply because there was nothing there to see. In fact, had the Data Scientist been developing some financial reports for the CFO, instead of a dashboard for the COO, the customer churn problem may not have been noticed at all. The just-so story could just have easily turned into an accounts payable fraud detection story instead. Most of all, the technology used to determine the customer characteristics could have been any product that was capable of the type of analysis the Data Scientist decided to try and was only utilised because of the unused license.
So you as the reader of the Use Case, and potential buyer of the vendor/software/technology, need to keep in mind the possibility that the story has been given to you in a “just so” form. This is particularly important as you will find that those companies that try to start a Data Lake promising to “increase sales by x%” or “decrease customer churn by y%” will be sorely disappointed. Despite the impression given by just so Use Case stories, Data Science is more akin to diamond mining than constructing a bridge. You need to add data capability to help uncover the gems that are no doubt there… but how big, how many and what type of diamond? No-one will know until you’ve uncovered the gems, a long time after the decision was made to invest.
Postscript: I’m aware there is a Gartner Report which fundamentally disagrees with the above (i.e. 90% failure due to uncertain use cases). However I submit the analysts may have fallen for the trap described in this article, looking at results after the fact. Were the 10% successes really designed specifically for the issue that eventually rendered them a success. Or were they they just the lucky 10% who found something. More likely the 10% figure itself comes from a Woozle Effect.